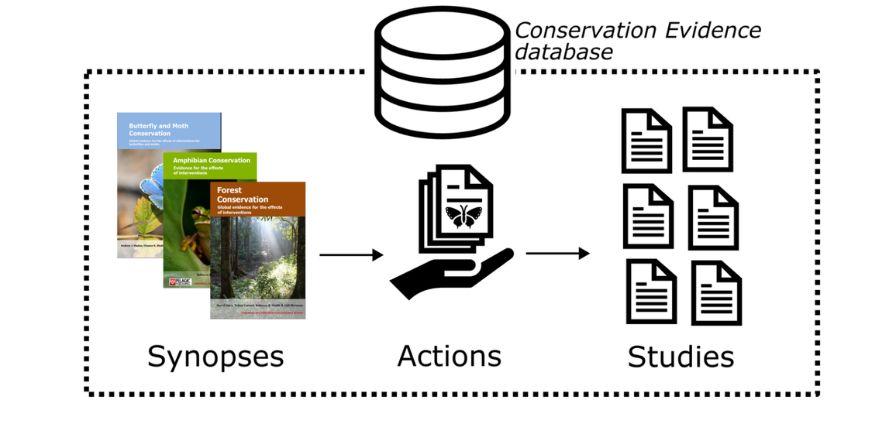
Submitted by Abigail Youngman on Fri, 16/05/2025 - 13:43
Making the right decisions to protect species and habitats is crucial, but it's tough when scientific knowledge on conservation actions is scattered across thousands of studies.
Even though resources like the Conservation Evidence database, contain searchable summaries of conservation actions and their outputs, finding the right information quickly is still a challenge. This difficulty means that research is not being used to its full potential to conserve biodiversity.
A team, led by Radhika Iyer, an undergraduate student here in Cambridge, funded through the AI@Cam initiative and the Undergraduate Research Opportunities Programme, set out to investigate.
They wanted to see if modern LLMs could accurately retrieve information from the Conservation Evidence database and answer simple questions, to compare how they stacked up against human experts. The paper is published today in the scientific journal PLOS One.
The study’s results show that carefully designed AI systems could act as expert-level assistants to access specific evidence from databases. Quickly pointing conservationists to the most relevant evidence to address their specific problem.
However, their findings also come with a strong dose of caution. Simply plugging a question into a general chatbot is not the way to get reliable evidence-based answers. The setup – particularly how the system retrieves information – is crucial to avoid poor performance and misinformation.
This study is a first step, future research needs to explore how well these systems perform on more complex questions requiring nuanced, free-text answers.
There are ethical considerations too, including: ensuring equitable access to AI expertise and resources; minimizing environmental costs by using the lowest cost, most efficient (ideally open source) models that give acceptably high levels of performance; and avoiding over-reliance on AI at the expense of critical thinking and evaluation of evidence.
Whilst AI won't replace the need for expert judgement and local, carefully built tools based on studies like this one could significantly speed up access to vital scientific evidence, helping conservationists make better-informed decisions for the future of biodiversity.
This text is a summary of a blog post for the Imperial College London website by Dr Alec Christie.
Read the paper: Iyer R, Christie AP, Madhavapeddy A, Reynolds S, Sutherland W, Jaffer S (2025) Careful design of Large Language Model pipelines enables expert-level retrieval of evidence-based information from syntheses and databases. PLoS One 20(5): e0323563. https://doi.org/10.1371/journal.pone.0323563
More about this research:
Dept of Computer Science and Technology news item: Can AI offer better conservation advice than human experts?